Artificial intelligence as a fortune teller: Rethinking fault detection and maintenance (predictive maintenance).
- Pascal Penava
- Oct 7, 2024
- 3 min read
The modern manufacturing industry is highly automated and dependent on machines that need to work reliably and without interruption. If a machine suddenly breaks down, this can result in expensive production downtime and repair costs. But what if machines could 'think ahead' to detect faults in good time and prevent problems before they occur? This is where predictive maintenance comes into play - a process that uses artificial intelligence (AI) and machine learning (ML) to detect faults at an early stage and recommend specific maintenance measures.
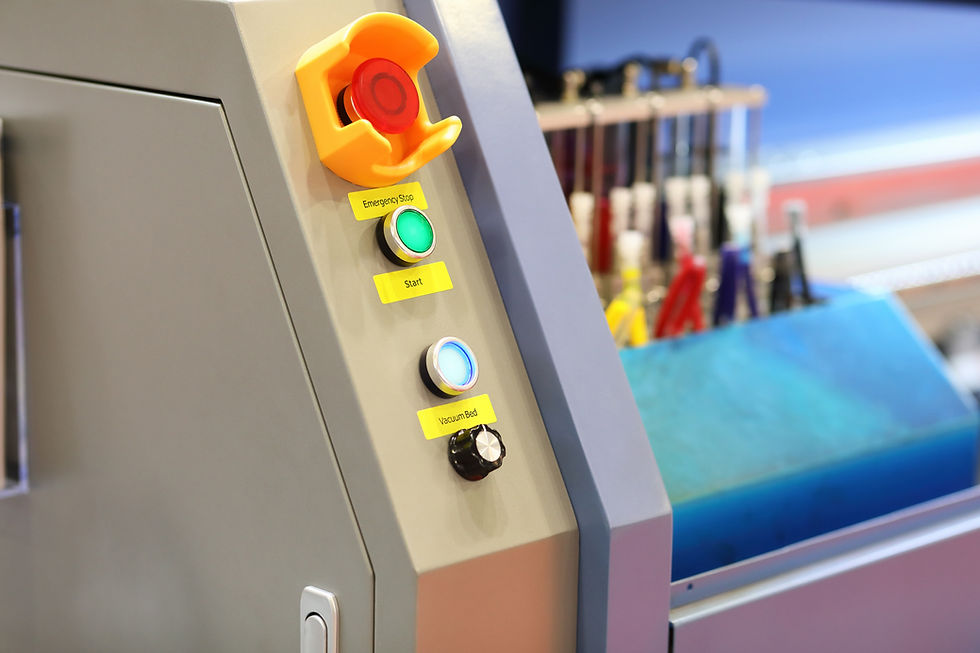
What is predictive maintenance?
Predictive maintenance (PdM) uses sensors that continuously collect machine data, such as vibration, temperature and pressure. This data is monitored in real time and analyzed using AI to identify anomalies and patterns that could indicate an impending failure. Unlike conventional maintenance strategies, such as preventive maintenance (where maintenance is carried out at regular intervals regardless of the machine's condition), predictive maintenance only intervenes when action is actually required.
How does predictive maintenance work?
The process of predictive maintenance begins with data acquisition. Sensors on the machines continuously collect information about their operating status. This data often comprises millions of data points that are analyzed by machine learning algorithms. Machine learning is a form of artificial intelligence that recognizes patterns by analysing historical data and makes predictions based on this. A commonly used method is the random forest algorithm, which works with multiple decision trees to classify the data and predict when a machine failure might be imminent.
A practical example would be the monitoring of an electric motor in a production plant. By using vibration sensors, the smallest changes in the motor's operating mode can be detected. The AI system compares this current data with historical data and recognizes anomalies that could indicate an imminent failure, such as uneven vibration. Before the engine actually fails, a warning is issued and maintenance is recommended - this not only prevents expensive repairs, but also minimizes downtime.
Performance and accuracy of the AI systems
The accuracy of predictive maintenance systems depends heavily on the AI technology used. Various studies have reported an accuracy of up to 85% in predicting faults, which is already a significant improvement over conventional maintenance methods. Some applications, such as wind turbine monitoring, have shown that AI-supported systems can detect faults in advance and thus extend the service life of machines by up to 10-20%. This precision makes it possible to monitor machines more closely and carry out maintenance measures in good time.
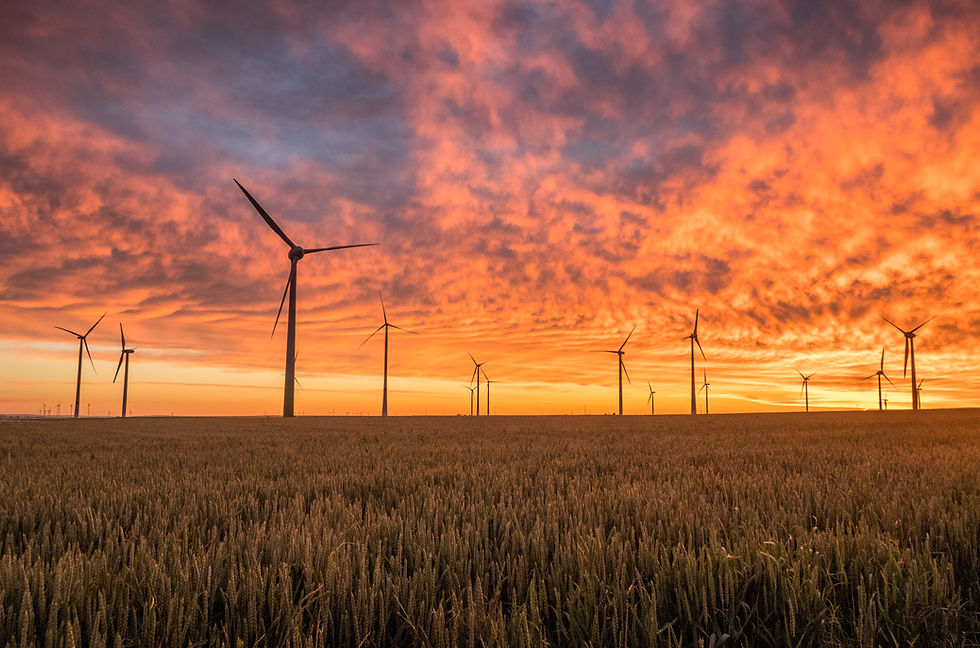
Advantages and challenges
Predictive maintenance offers numerous advantages for the manufacturing industry. One key advantage is the ability to save costs as potential machine faults are detected at an early stage. This means that expensive repairs and unexpected production downtime can be avoided. In addition, predictive maintenance ensures that downtimes are minimized as maintenance work is only carried out when it is really necessary. This increases efficiency as the machines run longer and more reliably. Another positive aspect is the optimal use of resources: companies can make better use of their machines as maintenance does not have to be carried out unnecessarily. Overall, this leads to higher productivity and more efficient production planning.
Despite these benefits, there are also challenges when implementing predictive maintenance. One of these is integration into existing systems, which requires careful planning. Companies need to invest in new technologies such as sensors and data collection systems in order to use predictive maintenance systems effectively. Another is data processing, as the machine data collected often includes huge amounts of information. This must be analyzed in real time, which requires powerful algorithms and infrastructures. In addition, it is important to train employees accordingly to ensure that they can use the new systems effectively.
Conclusion
Predictive maintenance with artificial intelligence is changing the way machines are maintained. By detecting problems at an early stage, expensive breakdowns can be avoided and the service life of machines can be extended. In a world that is increasingly reliant on efficiency and minimizing downtime, predictive maintenance offers a forward-looking solution for the industry.
References:
Carvalho, T. P., Soares, F. A. A. M. N., Vita, R., Francisco, R. d. P., Basto, J. P., & Alcalá, S. G. S. (2019). A systematic literature review of machine learning methods applied to predictive maintenance. Computers & Industrial Engineering, 137, 106024.
Comments